Jellytoring: a global tool in the making
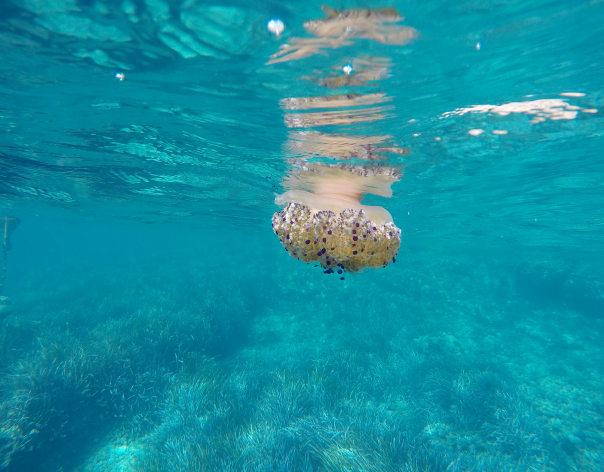
Figure 1. Cotylorhiza tuberculata, also known as the fried egg jellyfish
When encountering the gelatinous mass of a dead jellyfish on the beach it is often difficult to imagine how beautiful and graceful these creatures are in life. Although jellyfish are not quite as iconic as whales and dolphins, most people have some knowledge of these creatures, not least through the personal experience of being stung by a jellyfish on a seaside holiday.
The interaction that humans have with jellyfish, however, goes well beyond the occasional beach sighting or sting. In Southeast Asia some species of jellyfish are regarded as a culinary delicacy. Furthermore, jellyfish play an important role in traditional, as well as in modern, medicine. In particular, the venomous compounds found in the nematocysts (stinging cells in the tentacles) of some species are being researched for their potential as treatments for various forms of cancer.
Nevertheless, in opposition to these positive aspects, there are a multitude of negative effects jellyfish can have on human activities and wellbeing, especially when they occur en masse (jellyfish blooms). Jellyfish aggregations can clog seawater intake screens in water desalination and power plants, and cause significant socio-economic impacts by killing farmed fish in aquaculture facilities. They can interfere with commercial fishing operations by constituting a health hazard to fishermen retrieving their nets or through overloading the nets and spoiling the catch. And, of course, there are the negative impacts on coastal tourism.
Conversely, from an ecological perspective, jellyfish fulfil an important role in marine ecosystems as zooplankton predators and are themselves eaten by various marine organisms such as sea turtles, sunfish and spadefish.
Despite their ecological importance and wide global distribution, there are still considerable gaps in our understanding of these organisms. In particular we don’t know what influence current and future environmental change may have on their population levels. The combination of impacts from climate change, ocean acidification and overfishing has led to fears of a potential rise in jellyfish numbers, and a gelatinous future for our oceans.
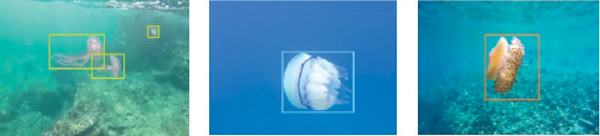
Figure 2. Examples of labelled training images where the instances have been marked with bounding boxes. From left to right: Pelagia noctiluca, Rhizostoma pulmo, and Cotylorhiza tuberculata.
Currently, scientists can neither confirm nor reject this scenario, as empirical data on adequate spatial and temporal scales is lacking. On a global scale, the lack of long-term data series is not unique to jellyfish, but in many ways the case of jellyfish data scarcity reflects the political and scientific challenges involved with establishing and maintaining such datasets. In particular, the long-term financial commitment and costs are key factors preventing the collection of long-term data globally. To date, data on jellyfish distributions have mostly been collected by means of visual observations from the shore or boats, often only generating snapshots in time on a local scale and requiring considerable numbers of personnel to collect and process the data.
Other collection methods such as the Continuous Plankton Recorder, although useful in collecting data over large spatial and temporal scales, are not ideal for jellyfish as they are easily damaged during the collection and preservation process. The availability of cheap commercial underwater cameras in recent years has facilitated the potential for replicated video observations, increasing the potential for collecting data on larger temporal and spatial scales. However, until recently, these video recordings needed to be processed manually by a human observer, involving considerable amounts of time and effort.
Advances in artificial intelligence, and in particular the advent of automatic computer-aided image classification using deep learning techniques, represent a regime shift for observational studies using cameras.
The integration of automatic image classification substantially reduces the sampling constraints related to the cost of data extraction and processing, and thus offers the prospect of easily moving beyond local scales.
A team of researchers composed of ecologists from the Mediterranean Institute for Advanced Studies (IMEDEA) and information scientists (University of the Balearic Islands, Spain), have successfully applied this technique in the development of a tool capable of identifying and quantifying local jellyfish species in real time. Dr Hilmar Hinz and Dr Ana Ruiz-Frau of IMEDEA formerly worked at the MBA (2005–2007) with Prof Stuart Jenkins (now Bangor University) and as part of the MarLIN team, respectively.
The team used deep learning by means of a convolutional neural network (CNN) to detect and classify different species of jellyfish from underwater video recordings.
Object detection is the procedure by which a new input image is analysed, and the position and classification of the target instances are identified, in our case jellyfish species. In humans, these types of classification skills are learnt during childhood and become effortless as we grow up.
For an artificial neural network to automatically detect and classify instances, it first needs to be trained with a large number of images where the objects of interest are labelled. This is usually done by a human observer who labels the instances by creating a rectangle or bounding box encompassing the object of interest (Fig. 2). This tells the neural network which pixels in the image contain the object, allowing it to learn its properties and characteristics such as colour, texture, aspect ratio, and so forth. This initial labelling step still takes considerable time, as several hundred pictures will need to be classified before the algorithm can correctly identify the object within a previously unclassified image or video sequence. Once the convolutional neural network is correctly trained, it can detect and classify images or videos in a fraction of the time taken by a human observer, often with much higher precision. When analysing a video sequence, the predictions can be forwarded into the quantification algorithm, which outputs the count of the instances of each jellyfish species over time.
The research team have named their jellyfish monitoring system Jellytoring (Fig. 3) and have recently published their results in the scientific journal Sensors.
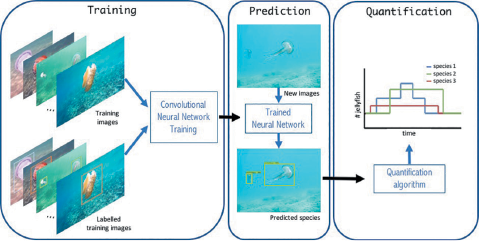
Figure 3. Workflow of the jellyfish monitoring system, Jellytoring
The system demonstrated outstanding performance on jellyfish detection, reaching accuracies of 95.2%.
Jellytoring also performed well on jellyfish quantification, correctly quantifying the number and class of jellyfish in real-time video sequences for 93.8% of their duration1. The results of this study are encouraging, opening up the possibility of inexpensive jellyfish monitoring systems, both to attain more robust scientific data on larger scales, and for use in applications such as early warning systems for beaches or the inlets of power or desalination plants.
The work presented in the journal Sensors included the detection of three species common in the Mediterranean (Pelagia noctiluca, Rhizostoma pulmo and Cotylorhiza tuberculata, see Figs 1 and 2). The research team is currently working on improving the performance of the tool and building a global Jellytoring application that can be hosted on a website to quantify jellyfish from uploaded videos or still images supplied by the public.
The team has now collected sufficient image material to classify up to 16 jellyfish species on a global scale. For the effective and accurate working of the tool, large numbers of training images are required. Here, the scientific community and the public can lend a helping hand. If you have underwater images of jellyfish (stills or video footage) and you are happy to share them with the researchers, please get in touch with the team through their dedicated email address jellytoring@gmail.com. The team is also looking for a business partner who would be interested in commercializing their concept through the design and construction of an autonomous monitoring system. The idea would be to construct an array of solar-powered buoys containing computers that can process the incoming video data from cameras located midwater on an anchoring chain or at the base. Generated count data would then be sent via a mobile phone connection to a land-based hub where the data would be further processed and visualised in near real-time. Such monitoring systems would have a wide range of applications as early warning systems for commercial operations and could also provide invaluable data for science to help close some of the remaining knowledge gaps in jellyfish ecology.
Hilmar Hinz (hhinz@imedea.uib-csic.es)1,Ana Ruiz-Frau (anaruiz@imedea.uib-csic.es)1, Miquel Martin-Abadal (miguel.martin@uib.es)2 and Yolanda Gonzalez-Cid (yolanda.gonzalez@uib.es)2
- IMEDEA–Mediterranean Institute for Advanced Studies at the University of the Balearic Islands (UIB), Spain
- UIB
Further reading:
Martin-Abadal, M., Ruiz-Frau, A., Hinz, H. and Gonzalez-Cid, Y. (2020). Jellytoring: Real-Time Jellyfish Monitoring Based on Deep Learning Object Detection. Sensors, 20:1708.
Graham, W.M., et al., (2014). Linking human well-being and jellyfish: ecosystem services, impacts, and societal responses. Frontiers in Ecology and the Environment 12(9) pp. 515-523
Lucas, C.H., Gelcich, S. & Uye, S.-I. (2014). Living with jellyfish: management and adaptation strategies. In: Lucas, C.H. and Pitt, K.A. (Eds) Jellyfish Blooms. Springer Netherlands, pp.129 150. https://doi.org/10.1007/978-94-007-7015-7
Martin-Abadal, M., Ruiz-Frau, A., Hinz, H. and Gonzalez-Cid, Y. (2020). The application of deep learning in marine sciences. In: Pedrycz, W. & Shyi-Ming, C. (Eds) Deep Learning: Algorithms and Applications. Springer,pp.193–230.
1An illustrative demonstration video can be found at http://srv.uib.es/jellyfish-quantification/